4-minute read
This article first appeared on the Utility Analytics Institute blog.
Quick summary: By using data to identify the areas at highest risk for vegetation-related incidents, machine learning platforms help utilities allocate resources where they are most urgently needed.
Vegetation management often represents a utility’s largest operations and maintenance cost, and with good reason. Trees are a leading cause of U.S. electricity outages. Controlling vegetation is a key factor in reducing wildfire risks. And North American Electric Reliability Corporation (NERC) maintains standards for maintaining clearance between vegetation and power lines (FAC-003-2), with fines for violations reaching up to $1 million per violation per day.
As the pressures on vegetation managers mount, the limitations of traditional approaches are becoming more apparent. Deploying inspection crews across the service area on a set schedule is not only costly and time consuming, but often gives no consideration to varying risk levels. Without a reliable means of evaluating and monitoring encroachment risk, utilities could be navigating dangerous territory where the possibility of vegetation causing an outage—or worse, a fire—is unacceptably high.
Data science and analytics enable utilities to tap into the power of artificial intelligence (AI) in managing vegetation for maximum safety and grid reliability. By using data to identify the areas at highest risk for vegetation-related incidents, machine learning (ML) platforms help utility companies allocate resources to areas where they are most urgently needed.
For many utilities, machine learning represents uncharted territory; fortunately, tapping into AI for vegetation management is not an all-or-nothing initiative. I’ve identified three levels of analytics application where utilities can leverage technology to deliver increasingly beneficial results.
Article continues below.
WEBINAR
How asset image analytics supercharges utility operations
WHITE PAPER
Transforming utility operations with asset image analytics
Level 1: KPI reporting
The first level of analytics-based vegetation management starts with understanding the utility’s own records of historical activities such as inspections, trims and audits. By summarizing this data in easy-to-consume reports, utilities can identify patterns around certain times of the year, certain regions, certain mixes of tree species, etc. These insights deliver enhanced visibility into current operations and help leaders identify opportunities to optimize how the work of vegetation management gets accomplished.
Level 2: Machine learning algorithms
If there’s one thing AI is good at, it’s analyzing huge amounts of data to yield decision-driving insights. Machine learning models can predict growth and risk levels down to the individual tree level, based on historic growth patterns, species, topography, weather forecasts and many other data points.
In determining what data is out there to leverage, LiDAR (Light Detection and Ranging) is becoming increasingly valuable to vegetation management. These systems send out light energy from a rapidly firing laser and measure the light reflecting off of the ground, trees and plants to create a 3D representation of the landscape. LiDAR enables utilities to gauge overall vegetation density, tree strike potential (the height of a tree compared to its distance from a power line), and normalized difference vegetation index (NDVI) to measure vegetation health.
As effective as LiDAR can be, one of its shortcomings is that, due to the high cost of scans and the reliance on external platforms such as aircraft, they tend to occur intermittently, so the latest data could be months or even years old. Satellite imagery data is another valuable dataset to utilize because images of the same point can be captured at a more frequent rate.
By identifying and leveraging various data sources that may be available, machine learning models can assign a holistic risk score to different trees, spans and/or circuits. These risk scores provide leadership with a valuable tool to drive their operational planning for different levels of their service territory.
Level 3: Data-driven operations
Level 3 combines data-driven understanding, machine learning model output and business understanding to effectively drive operational planning for risk reduction. It also looks to feed information back to inspectors for faster decision-making in the field. This data-driven approach helps utilities optimize the return on their labor costs because trucks can be deployed to highest-risk areas at a more frequent cadence and less often to areas at lower risk for vegetation-related outages or ignitions.
This level also enables communication back to teams in the field. Vegetation inspectors use tablet apps to document the results of their inspections and actions taken. Analytics platforms can integrate information from Levels 1 and 2 and deliver analytics back to field teams in real time. Instead of relying solely on their observations in-the-moment, vegetation inspectors can gain a more holistic understanding of the area, enabling faster and better-informed decision making while they are on site.
This is just one story highlighting the enduring need for “humans in the loop” when AI is implemented. Not only need employees not fear broad-based job cuts, but they also have the opportunity to broaden their resumes with skills such as managing AI bots, exploring new use cases, and implementing best practices.
A new era in preventing vegetation-related issues
Effective vegetation management enables utility companies to protect their assets while preventing encroachments that could lead to outages or fires. By leveraging the unique advantages of analytics and machine learning, vegetation managers can automate the gathering and analysis of the data necessary to make the most efficient use of their resources. Highest-risk areas can be prioritized for proactive maintenance, helping utilities ensure that power keeps flowing while protecting the local environment.
Asset Image Analytics
Logic20/20 leverages the latest in machine learning and AI to develop impactful asset image analytics solutions for:
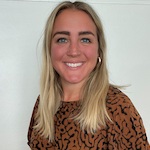
Frederike Dubeau is a Manager in Logic20/20’s Advanced Analytics practice.