5-minute read
Quick summary: Explore the critical steps for evolving from enthusiasm to true generative AI readiness through practical measures in business strategy, IT strategy, and AI governance.
Generative AI capabilities have grown by leaps and bounds, capturing the imagination of business leaders. The free chat interface of ChatGPT has introduced this new mode of machine learning to millions. Executives are pushing their organizations to take advantage and innovate ahead of competitors. Meanwhile, employees are clamoring for access and the ability to use it to aid their day-to-day work.
It sounds like a win-win. If executed well, generative AI has the potential to speed up developers, automate processes, and create innovative new capabilities. However, if organizations don’t prepare appropriately, it can lead to spiraling costs, unsupportable business operations, misuse, and cybersecurity vulnerabilities.
Mature organizations procure software with clear business cases and standard IT controls. It is useful to think of generative AI in a similar way, but with a few unique features that require care. Specifically, Gen AI adoption should be prepared for with a business strategy, technical controls, and AI governance.
Business strategy
Do you have defined goals and use cases for AI?
First and foremost, it is important to have clear objectives for generative AI adoption. Do you want to boost productivity? Reduce costs? Improve customer experience? Gain a competitive advantage? There are many use cases to explore, but focusing first on the outcomes can help narrow the focus of your initiative. Even if it is purely a research and development effort, it is good to set goalposts and timelines for what you hope to achieve by adopting generative AI.
Is there a business case for generative AI?
There are multiple ways to use and deploy the technology, each of which comes with its own cost structure. For example, APIs may charge by “token” (words or fragments of words), but tools like Copilot may charge by user. Custom deployments may improve your ability to use advanced models, but can come with expensive hardware requirements.
Action items to take in this area include the following:
- Model your expected usage patterns, user base, and total cost before you get started.
- Make sure you have good cost monitoring in place so there are no surprises.
- Ensure that you are within a budget that is justified by the expected use cases.
- As with any initiative, track your business impact! Set KPIs for the target use cases and measure them to ensure the business is getting enough value out of it to outweigh the expense.
Do you have a change management plan for AI adoption?
Generative AI is exciting because of the new capabilities it brings and the flexibility it has to be used in many scenarios (code development, copy editing, summarization, knowledge base search, customer support, etc). Each use case has different impacts on business users and their processes.
Preparing users to understand the capabilities, the limitations, and the practical steps for using generative AI is important to successful adoption. If you have governance processes in place (as we recommend below), it is also critical for business units to understand the costs and governance steps for accessing generative AI tools.
IT strategy
How will you control access, cost, and standardization of AI tools?
ChatGPT, DALL-E, and other publicly available AI tools have reached millions of people. Models are improving constantly and developing new multi-modal capabilities. It is an exciting, innovative space, but it is important from an IT perspective to control and standardize generative AI tools in the business. Although generative AI is new, it should be provisioned like any other software to avoid messes that can be created if every user goes their own way in adoption. The result would be many unsupportable tools, duplicative costs, and security issues.
Governing AI software allows controls over cost, security, and safety, to name a few. Meeting the excitement of potential users without slowing down requires identifying the tools that best suit the business and providing a clear procurement process. Additionally, it is important to have user feedback channels and pilots to explore new AI tools as they appear in the market. A successful IT AI strategy stays ahead of the users, bringing new tools as they come online.
Gen AI should be provisioned like any other software to avoid messes that can be created if every user goes their own way in adoption.
AI governance
How will you ensure safe and effective usage of AI?
Generative AI’s capabilities bring with them a combination of old and new risks. Already there have been many public instances of generative AI gone wrong, including leaked customer data, false legal filings, damaging customer experiences, and biased automations. Employing generative AI safely to avoid these issues is not simple, but a good process can make it achievable.
We recommend an AI governance framework that addresses each risk with a clear process. The key risks in the AI governance framework are
- Privacy
- Ethics
- Legal & compliance
- Performance
- Operations
- Security
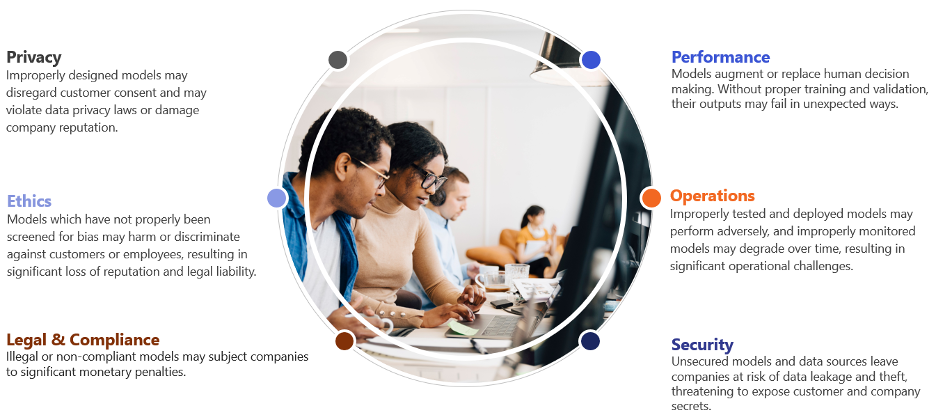
A framework for the future
Employing this framework gives business units clear definitions for the requirements of safe operation of AI. When paired with guidelines and a governance process, it can be streamlined so that business users can focus on applying this exciting new capability to solving problems and meeting objectives.
In a future blog post, we will show how to execute an AI governance program. Stay tuned!
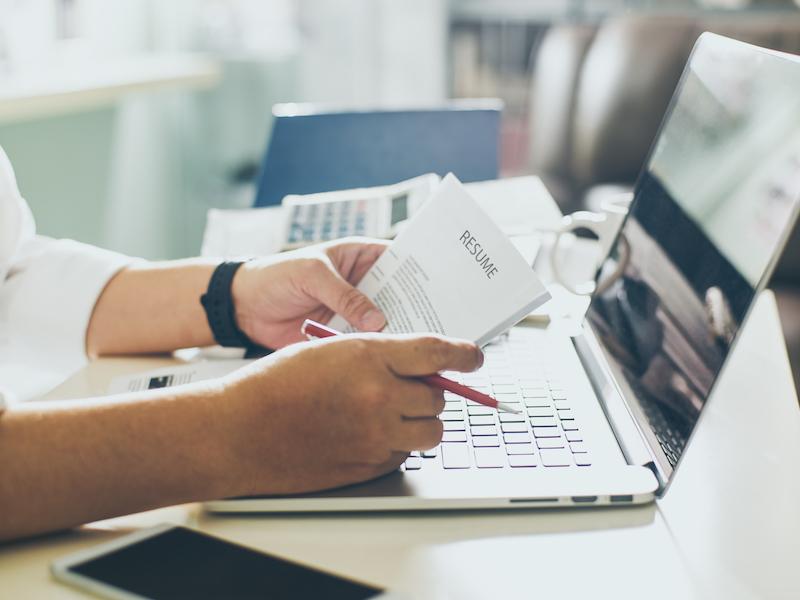
Put your data to work
We bring together the four elements that transform your data into a strategic asset—and a competitive advantage:
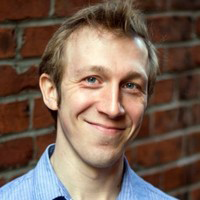