3-minute read
Data mesh is a very popular concept that’s making waves around industry conferences, blogs, and videos. It’s both a referential data architecture and a business operating model, and some people will describe it with names such as data fabric or domain-driven data modeling. Everyone is talking about “doing data mesh,” but companies often fail to reach its true potential because they don’t understand how all the components must work together.
How we got here
The core of data mesh comes from the long tradition of moving analytics from centralized to decentralized operating models. Traditional data warehousing started as a centralized approach where the IT team owned all aspects of analytics. This led to robust standards and data governance, but left many end users waiting for data.
The next major change was the data democratization and self-serve movement that gave end users more access. This allowed IT to focus on ingesting and sharing data sets, but led to an unruly mess of dashboards, cubes, and unregistered data sets, leading to more confusion as each business group derived conflicting insights.
This worked great until the “big data” movement, where streaming, semi-structured data required new concepts such as data lakes to manage. This provided all the data people needed, but made it very difficult for analysts to make sense of the data marsh.
The next movement focused on enabling data literacy with training and new tools. This helped but didn’t really address the goals of flexibility, quality data, and ease of generating insights.
Aligning to an operational North Star
Data mesh can be accomplished with at least two different approaches:
1) Embedded teams with automation: Distributed domain-based data teams create and publish data products critical to their domains while the central IT/data team provides tools to support automation and standards.
2) Product management with data teams: Data teams create data products but coordinate with domain experts and data producers on business context and data quality.
Is your team ready?
While the potential for data mesh is tremendous in improving analytics quality and increasing speed to insight, many pre-requisites are needed. The beauty of data mesh is that it brings together all the great advances in the analytics world:
• Product management
• Agile + DataOps (based out of DevOps)
• Contextual master data management
To fully achieve the benefits of data mesh, you need to be at a minimum maturity level in each of the following areas. If not, the overall process will get blocked and fail to achieve data mesh’s true potential.
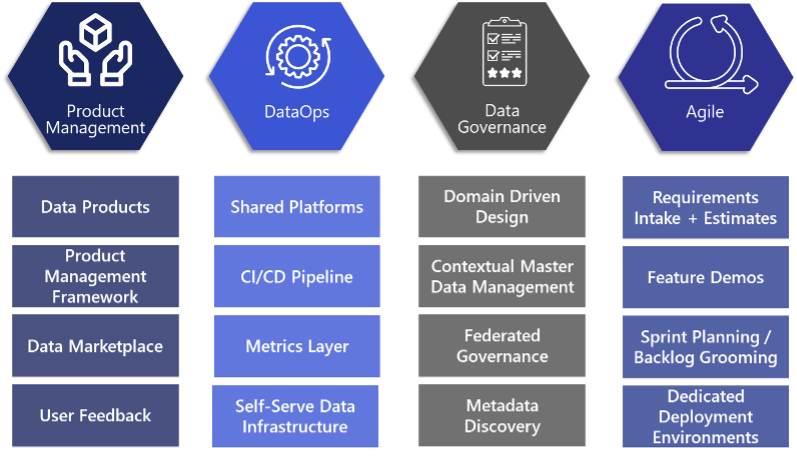
Product management
Teams need to be able to define, develop, and launch data products, which requires understanding their marketplace and users. This includes a robust management framework that can capture user feedback and a method for data users to access data products via a marketplace.
DataOps
The central data team needs the capability to enable shared platforms that include automated metadata discovery. The true goal is to automate everything not directly connected to insight development and remove friction for data product teams.
Data governance
The enterprise needs to be leveraging people, processes, and technologies to run a truly federated governance program, pushing data quality closer to data creators using a domain-driven approach.
Agile
Data teams need to be well-versed in Agile management principles to quickly pivot and delight customers.
Once these prerequisites are in place, your teams will be ready to move forward in pursuit of data mesh. excellence.
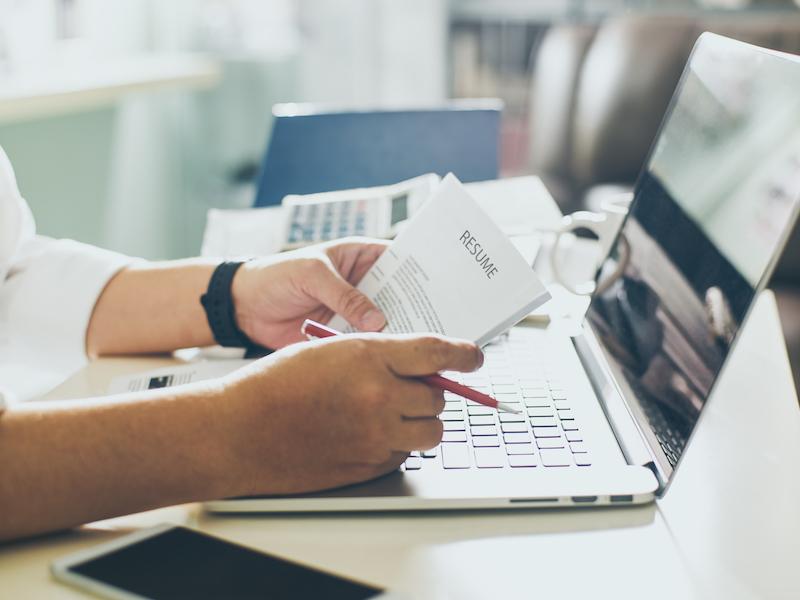
Put your data to work
We bring together the four elements that transform your data into a strategic asset—and a competitive advantage:
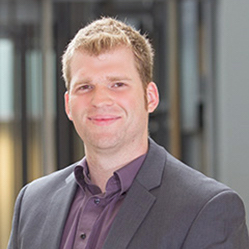
Mick Wagner is a Senior Solutions Architect in the Advanced Analytics practice at Logic20/20.