3-minute read
This article originally appeared on LinkedIn.
Quick summary: Struggling to grow adoption of your data marketplace? Borrow some insights from the pros in retail customer service.
One of the biggest challenges in data analytics is getting user adoption.
By creating an enterprise data marketplace as part of your greater data strategy and data mesh, you make it easier for more people to use your data to create analytics.
Common challenges in building a data marketplace include
- The complexity of the data
- Data quality
- Understanding the data lineage
- Your team’s current comfort with using data (or data literacy)
When I address the challenges of adoption, I like to review our First Foundation principles.
One of the first principles we always estalish is to meet your team where they are. Not every team will have a collection of data savants ready to devour data. Some folks use data as a secondary responsibility to their core jobs.
A complementary first principle is to reduce friction in getting results. Analytics is hard enough, and no one wants to have to scrub data and figure out infrastructure.
When building around these principles, we like to pull from design thinking concepts; in this case, know your audience and design around their analytics persona.
First principles to adoption
- Meet your team where they are.
- Reduce friction in getting results.
- Know your audience and design around their analytics persona.
To reduce friction and tailor to different personas on our team, our data marketplaces will pair a data catalog with three tiered customer service levels: self-serve, inspired, and guided.
Lessons from retail customer service
I like to compare this to shopping at a retail store, for example, Nordstrom.
Here’s how Nordstrom and a well-managed data marketplace do customer service:
Self-serve
I can pop into Nordstrom, look at the directory, go to right where I need to go, and grab a new pair of jeans and a shirt.
For a data marketplace, this is like a data scientist using a data catalog, searching for revenue data, and then connecting to the finance team’s API to use their data.
Inspired
When I’m in Nordstrom, I see a stylish mannequin wearing a vest with a tartan scarf. Brilliant—never thought to combine the two—so I pick them up on my way out the door.
For a data marketplace, we will create “starter kits” so that people have an interesting collection of data integrated, with some KPIs defined in a Power BI workbook (e.g. customer, sales, and marketing data).
Guided
I get home and my wife tells me to return all my choices. I return to Nordstrom and get a personal shopper to help me build a new wardrobe that makes me (more) stylish. I get outfitted with new jeans and a new shirt, and then I’m guided to a tailor who can make sure they fit perfectly.
For a data marketplace, a white-glove service team works with users to understand their needs so they can create a bespoke set of analytics. This tends to lead to a new data product such as a dashboard or data science predictive analysis.
There are many ways to approach adoption, and retail customer service provides inspiration on how to make it easier for your customers to use data.
It’s all about user experiences
There are many ways to approach adoption, and hopefully, the scenarios above will give you some ideas on how to make it easier for your customers to use data.
If your team is struggling to get adoption with your data marketplace, take some time to think about the end user’s experience. Spending 30 minutes shadowing your customers will teach you much more than telemetry data.
How can you make it easier for people who are not like you to use your data?
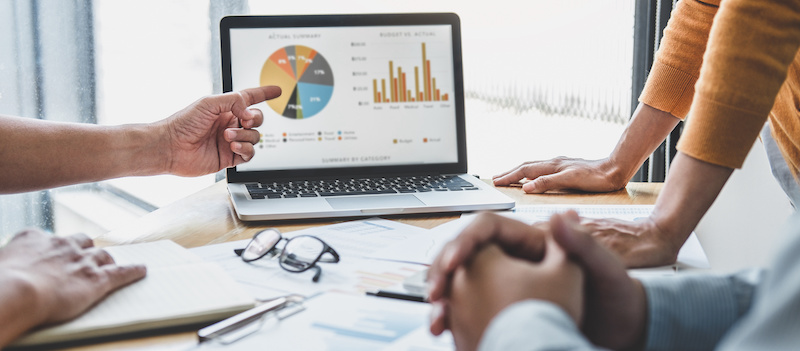
Put your data to work
We bring together the four elements that transform your data into a strategic asset—and a competitive advantage:
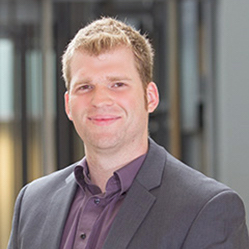
Mick Wagner is a Senior Solutions Architect in Logic20/20’s Advanced Analytics practice.