Snapshot
Safety is the top value of one of our California-based utility clients. As part of their state-mandated Wildfire Mitigation Plan (WMP), the utility needed to provide additional data insights to prove that actions taken within their vegetation management program were effective. We used statistical methods and created a machine learning model to analyze impacts of their vegetation management program. This included historical vegetation data, thorough documentation, and a white paper that enables our client to demonstrate how their program meets WMP requirements.
We brought our expertise and experience in:
-
Analysis which included exploratory data analysis, statistical tests, and a new machine learning model
-
Documentation of model methods and code
-
Whitepaper analysis that provided findings of effectiveness of vegetation management activities
Power across the West
Our California-based utilities client supplies natural gas and electricity to over 3 million customers every year. Since incorporating over 140 years ago, this Fortune 500 company has built more than 10,000 miles of underground power and 6,000 miles of overhead power.
Modeling for WMP compliance
Our client needed a reliable, data-driven approach to confirming the effectiveness of their vegetation management program, specifically in reducing vegetation-related outages. Their available dataset included factors like line clearance distance, genus, and location of trees. If analyzed properly, this data could reveal an archetype for high-risk trees and provide information necessary for a data-driven, actionable wildfire mitigation plan.
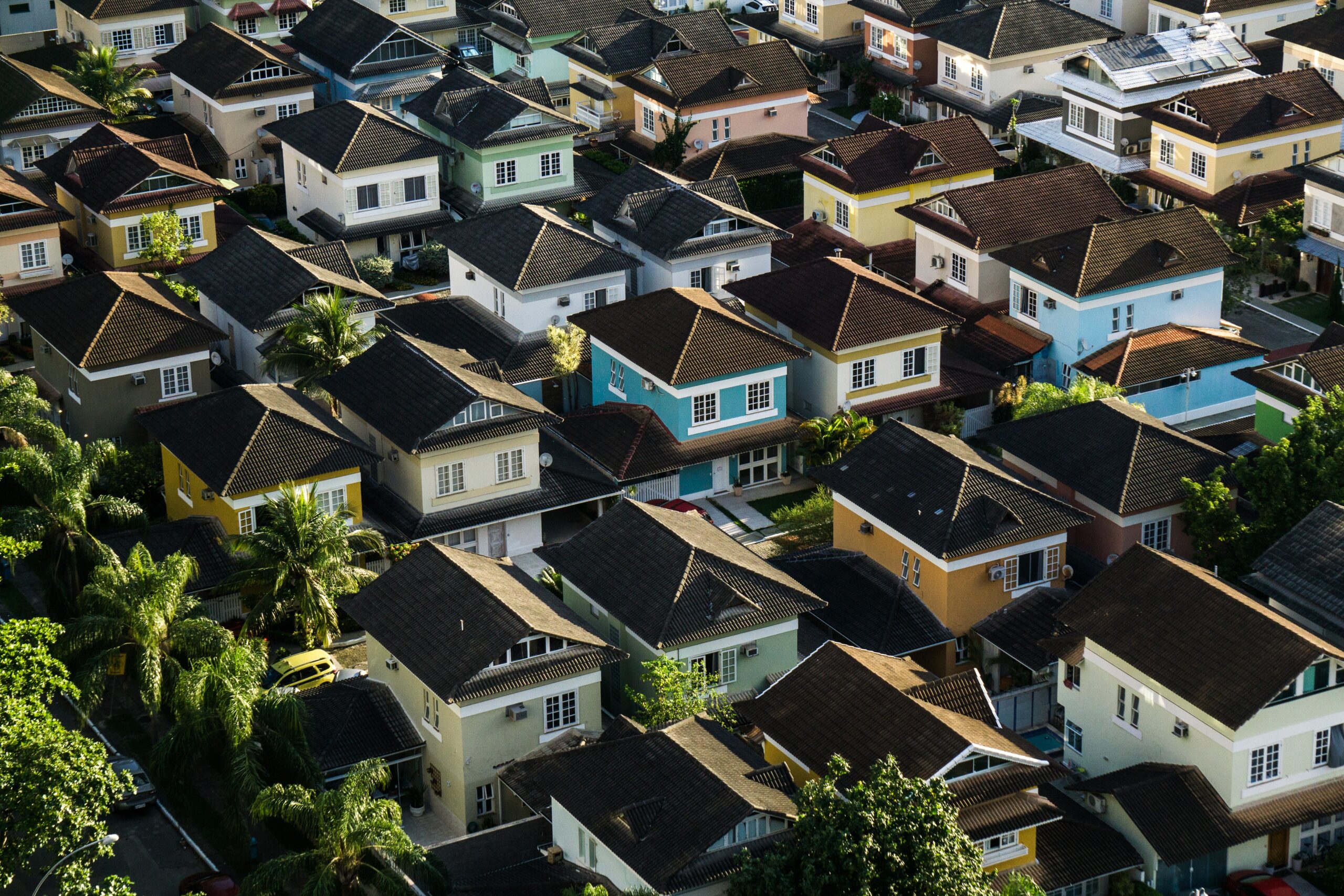
The power of machine learning
To help our client prove their wildfire risk management and preparedness, we analyzed over 12.5 million records.
Our process involved four steps:
1. Compile holistic vegetation history using data from their work management system that included activities related to inspection, trimming, and audits.
2. Flag vegetation related outage events from this historical data and tie it to the last activity
3. Train machine learning models to assign risk scores to all individual trees.
4. Use this data and model to:
a. Conduct sensitivity analysis to show level of impact enhanced clearance distance has on outage events.
b. Understand the different levels of risk associated with particular species.
c. Conduct counterfactual analysis to estimate the outage events prevented from enhanced clearing program.
We also validated the results of our analysis, proving the reliability of the client’s dataset.
Minimizing future risk
We provided an in-depth evaluation of which factors are important for understanding vegetation management and risk reduction. We used quantitative modeling to identify high-risk trees and show the impact of current trim clearance programs. We also provided documentation of the methods and codes used in our models.
This information has had an immediate, positive impact on our client’s vegetation management program. The outputs from our analysis allowed our client to document their management of wildfire risk in their 2022 Wildfire Mitigation Plan. There is additional work being done to use the tree risk model to prioritize and develop an optimized inspection schedule, helping our client plan for future risk mitigation more effectively.